Mathematics Professor Awarded Patent for Medical Imaging Project
Lixin Shen's project involves emission computed tomography reconstruction
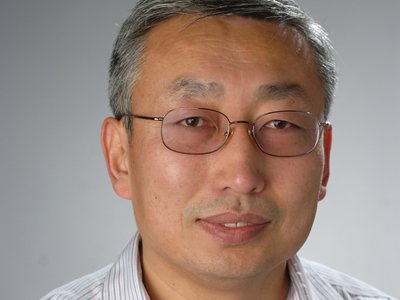
A mathematics professor in the College of Arts and Sciences is the co-recipient of a patent for a project that enhances medical imaging research.
Lixin Shen, an expert in image and signal processing, is part of a quartet of researchers whom has been issued a patent by the U.S. Patent and Trademark Office. Their project is titled “Methods and systems for inverse problem reconstruction and application to ECT [emission computed tomography] reconstruction.”
The other recipients are Andrzej Krol, professor of radiology and associate professor of pharmacology at SUNY Upstate Medical University; Yuesheng Xu, professor emeritus of mathematics at Syracuse; and Si Li, a former visiting scholar at Syracuse.
Xu and Li are mathematics professors at Zhongshan University in China.
“This is a huge honor for Professor Shen and the mathematics department, in general,” says Uday Banerjee, professor of chair of mathematics at Syracuse. “This patent supports medical imaging research throughout Central New York, while improving the accuracy of clinical decisions. It exemplifies the spirit of collaboration and entrepreneurship that permeates our region, particularly between Syracuse and SUNY Upstate.”
ECT is a method in which a three-dimensional object is represented by a series of two-dimensional slices. Popular in the medical field, ECT relies on non-invasive radiographic techniques to produce thin, cross-sectional views of organs and tissues. The images are then used to diagnose abnormalities or diseases.
Central to the process is mathematics.
“Ever since doctors starting using X-rays at the beginning of the 20th century, medicine has relied heavily on different imaging techniques,” Shen says. “Today, these methods use either an internal or external source of radiation.”
Most people are familiar with the latter modality, thanks to X-ray and ultrasound methods, in which radiation is detected after it passes through the body. “The result is an image that forms from the way the radiation is absorbed by the body,” he continues. ECT, however, exemplifies the internal modality, involving a source from inside the body. “The source is usually a radioactive drug, or tracer, that is injected, swallowed or inhaled by the patient, depending on what is being studied.”
The mathematics behind tomography were worked out in the early 1900s; however, it wasn’t until some 60 years later, when the first practical scanning device was created.
Since then, tomography has touched on almost every branch of science and technology, including physics, chemistry, astronomy, geophysics and medicine.
“ECT works because of a combination of precise measurement techniques, sophisticated computer algorithms and powerful mathematics,” Shen says. “In medicine, ECT provides us with three-dimensional functional information, as opposed to simpler structural information you might glean from a CT [computerized tomography] scan or an MRI [magnetic resonance imaging] test.”
Clinical applications of ECT include detecting, staging and monitoring responses to cancer therapy; detecting and stratifying cardiovascular diseases; mapping regional blood flow in the brain; and scanning bone, lungs and kidneys.
Convinced of the need to reduce the amount of radiation people have been exposed to during ECT examinations, Shen and his colleagues originally tried lowering amount of activity in the tracer. The only problem was that it led to a high amount of shot noise—electronic noise stemming from an electric current—in the raw ECT data.
“Noisy data, if treated by conventional techniques, leads to very noisy and clinically unacceptable reconstructed images,” he says.
Shen and his colleagues responded by approaching ECT as an optimization problem—finding the best solution from all feasible solutions. Using a preconditioned alternating projection algorithm (PAPA), they have been able to better determine the radioactivity distribution in the patient and achieve higher-quality images.
“Based on performed numerical experiments, PAPA performs well in terms of the quality of the reconstructed images,” Shen adds. “It provides better structure elucidation and improved feature visualization, thus helping us solving key biological problems. … It’s our hope that this process will help revolutionize medical imaging analysis—proof that math can save lives.”